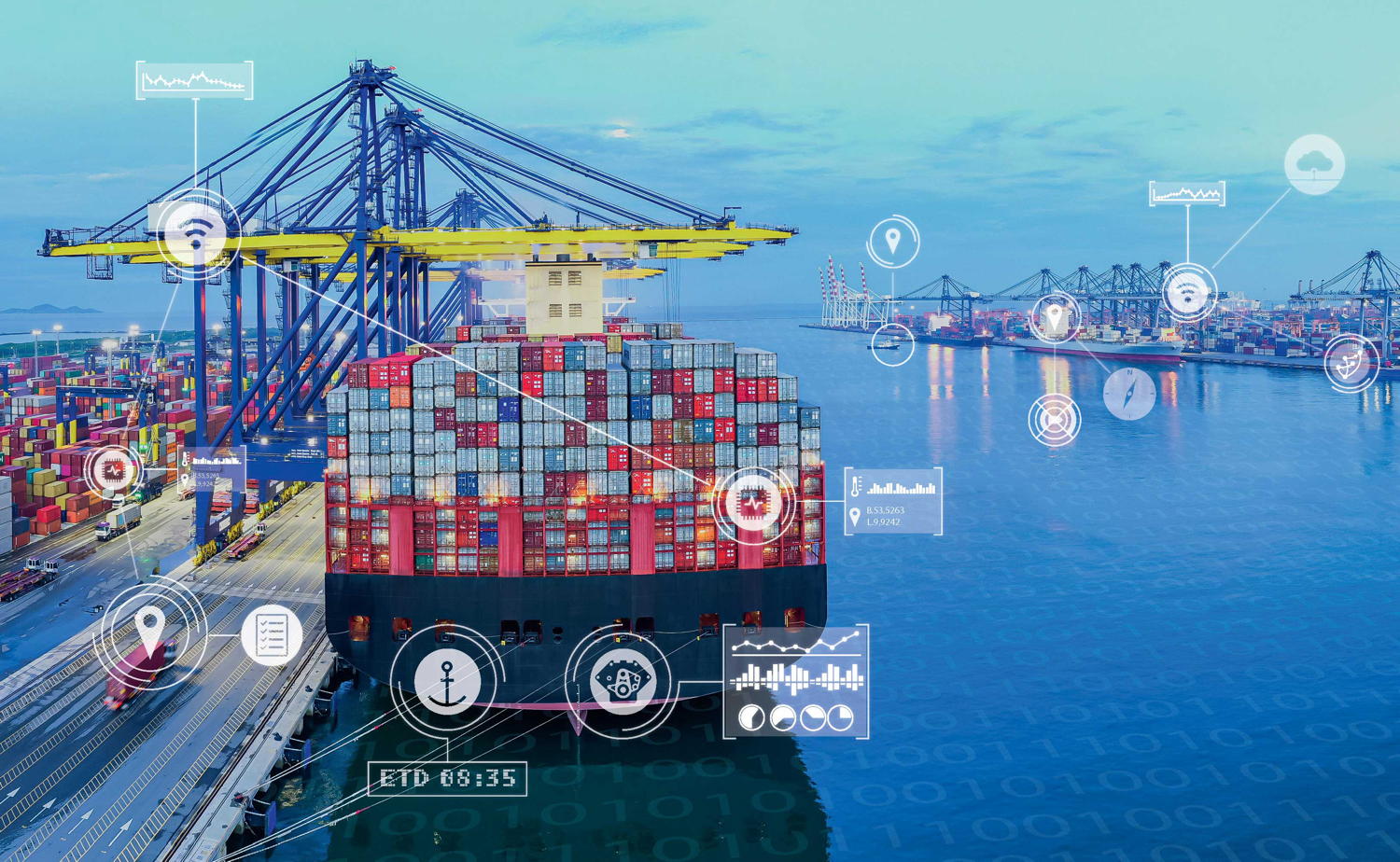
Hamburg-based Fraunhofer Center for Maritime Logistics and Services CML has published a new White Paper »Machine Learning in Maritime Logistics« analyzing potentials and use cases
Digitalization initiatives by ports and shipping companies in recent years – driven by the obligation to equip AIS transmitters or through[ds_preview] better maritime connectivity – have created a unique maritime, continuously growing database. In order to make smart use of this data and maintain competitive advantages in all segments of the maritime industry, machine learning (ML) is seen as an important means, allowing companies e.g. to:
• Reduce costs through optimized operations and data-driven decision making,
• Improve quality control and safety through digital monitoring solutions,
• Capture knowledge hidden in past business records,
• Identify decision-relevant information in large data sets,
• Automatize processes using intelligent assistants, and
• Establish new business models and products.
But what exactly is ML and why is it still not utilized widely in the marine world, even though everyone is talking about it?
Not a magic bullet
Machine Learning is a subset of artificial intelligence, a wide field in computer science concerned with equipping machines with human intelligence. With ML, rules for obtaining answers from data are not manually programmed (as done in traditional planning algorithms, e.g. for assigning port equipment to waiting containers) but the outcome of the ML algorithm itself.
These rules are automatically generated in a training phase and then applied to new data to derive answers for a specific, clearly defined problem. Therefore, ML can be interpreted as an advanced form of classical statistics, in which knowledge is also inferred from data examples. That being said, ML can only learn based on the information actually hidden in data available in a given use case. Unfortunately this means, if data is not substantial enough, ML is not going to offer a solution for a problem at hand.
Powerful but not universal
There are three main types of ML, supervised, unsupervised and reinforcement learning, each with a broad variety of algorithms, application areas, strengths and weaknesses. While supervised ML is trained with data where inputs and corresponding outputs are known, unsupervised ML works on unlabelled data to identify patterns within a data set. Alternatively, reinforcement learning discovers an optimized strategy for decisions problems via a sequence of trial and error experiments.
What all of these algorithms have in common is that they require a certain minimum of high-quality data, and usually involve a careful pre-processing of this data. Also, the scope of the problem to be solved, i.e. the use case, has to be manageable and suitable for the given database. Thus, defining the use case is an important and challenging first step in all ML projects.
Not necessarily disruptive
With ML you can »start big or small«, but based on our experience it is recommended to start with proof-of-concept applications instead of going straight for the big all-embracing leap. ML can be a complement to methods already in use. As a rule, it makes sense to start with a proof of concept get it working and then expand the scope step by step to other areas over time.
Far from exhausted its potential
ML has shown great success in many areas such as image or text recognition, healthcare applications, recommender systems, or robotics. In the maritime sector, ML has been e.g. used to estimate arrival times or for autonomous navigation, condition-based monitoring and route optimization. But although data-driven decision-making is recognized as a key to success, a majority of companies has not yet started exploiting ML in its different forms. According to a recent study, only 20% of German logistics companies are using big data analytics and merely 6% already take advantage of AI technologies. Meanwhile, 90% regard AI as very important to simplify business processes and increase productivity in the transport and logistics sector.
What is the reason for this discrepancy between perceived importance and actual use? Especially for small and mid-sized companies the implementation of ML projects can strain available personnel, time, or financial resources. However, more often the shear amount of possible applications, algorithms and data sources can be difficult to navigate for ML beginners. Each algorithm has individual strengths, fits certain fields of application and requires careful tuning of parameters during training, all of which requires specific knowledge and sufficient experience. Thus, success in the field is by no means a sure thing but requires careful selection of suitable use cases and pooling of necessary expertise either inhouse or by involving external partners.
Nonetheless, our answer to the question »Machine Learning – just a buzzword?« is a clear no. Without being a panacea, ML carries the potential to impact many business processes, increase efficiency and reduce workloads. How early adaptions of ML look like in the maritime sector, e.g. to provide reliable ETA predictions or fuel consumptions forecasts, is shown in the white paper. And once the first hurdle has been cleared, ML will find many more successful applications in everyday operations of maritime companies and SMEs.
White Paper »Machine Learning in Maritime Logistics« Available for download at www.cml.fraunhofer.de
Miriam Zacharias, Steffen Klöver, Lutz Kretschmann Fraunhofer Center for Maritime Logistics and Services CML